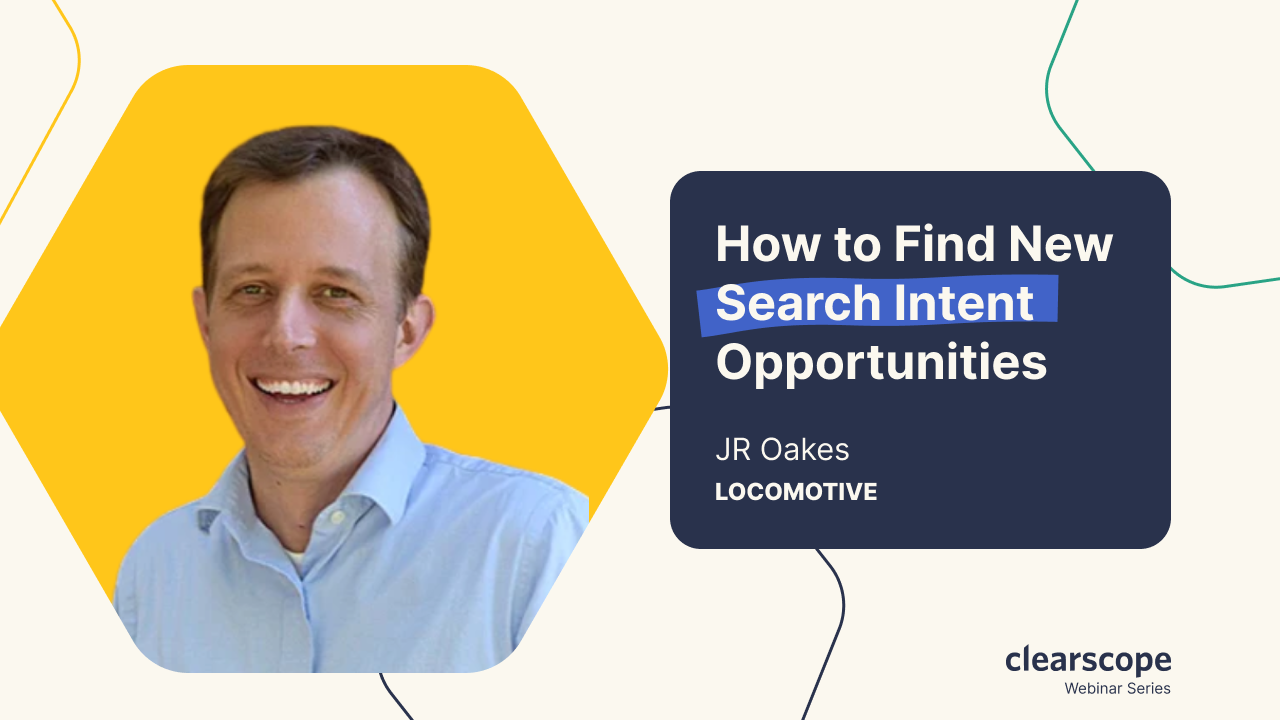
Webinar recorded on
Join our weekly live webinars with the marketing industry’s best and brightest. It’s 100% free. Sign up and attend our next webinar.
Join our newsletter
Get access to trusted SEO education from the industry's best and brightest.
JR Oakes of LOCOMOTIVE stopped by Clearscope to share how to find new search intent opportunities.
In less than an hour, JR covered:
What search intent is.
Problems that an intent-focus solves.
Limitations SEO’s have with an intent-focus.
How intent aligns with your user journey and funnel.
Organizing the current intent that your site covers.
Strategies for finding new intent (“whitespace”) areas to cover.
Here's our biggest takeaways from JR’s talk:
Keywords are not intent. You need to map intent to keywords.
Align search intent with your user journey and funnel for success.
Strategies for finding new intent areas to cover. Mine new opportunities with ValueSerp. Then categorize the search queries and measure the certainty of the classification with Nemo.
And check out the resources JR shared below:
Flair and Spacy: Converts phrases like Raleigh, NC to LOC (NER), lemmatizing, and filtering very common or rare words.
ValueSerp: API for Google results.
NeMo: A tool to measure the certainty of the classification predictions.
Watch the full webinar
About JR Oakes:
JR Oakes is the VP of Strategy for LOCOMOTIVE Agency. He has been an SEO since 2011 and was formerly an architectural glass artist. His focus areas are in machine learning, language, and user experience.
Follow JR Oakes on Twitter: https://twitter.com/jroakes
About LOCOMOTIVE Agency:
LOCOMOTIVE Agency is a full service SEO agency with an intense specialization in Technical SEO, many-location Local SEO, dynamic language insights and generation at scale using state-of-the-art language models, and consulting on extremely large websites (100M-2B+ URIs) with sectional / topical content analysis, mass scale internal PageRank multi-variate analysis, and more. Our clients range from the Fortune 500, to the U.S. Military, startups, and SMBs.
Follow LOCOMOTIVE Agency on Twitter: https://twitter.com/LocomotiveSEO
Read the transcript
Bernard:
Awesome. Well, I'd say let's get this started. We have JR Oakes here. JR Oakes is the VP of Strategy for LOCOMOTIVE Agency. He has been in SEO since 2011, and was formally an architectural glass artist. His focus areas are in machine learning, language and user experience. LOCOMOTIVE is a full service SEO agency that does a lot of amazing work in services involving deep machine learning.
Bernard:
They work with lots of local companies, lots of very large websites, and their clients range from Fortune 500 to US Military, startups, and SMBs. We are super excited to have JR talking about how to find new search intent opportunities. He's pulled me over and over. It's going to be very nerdy, very geeky. And maybe even, I'm not going to understand some of the things that he has to say.
Bernard:
But if you have questions, we want to wait until the end of the presentation to get those answered. But please drop them into the Q&A. So that when it comes across your mind, you can just put it there and we will get to it at the end of the presentation. JR, the stage is all yours.
JR:
Well, first off, thank you, Bernard, you and Clearscope for having me. I really enjoy talking about this stuff. So hopefully everybody finds it valuable. So the title of the presentation is, How to Find New Search Intent Opportunities. If I can make this about me, Bernard, whenever, some of this, I'm a big open source and tech geek. So follow me on Twitter. You can also follow me on GitHub, if that's your thing, same username. And then our website is locomotive.agency. We used to be Adapt Partners back in the day for those that know that.
JR:
All right. So what is intent? Intent is something that I have heard for the last five years, six years. And I think there's no agency or article that doesn't mention intent in some way. I think that's the thing that Bill Solosky used to talk about things not strings. What is the thing people are looking for? That's what we're going after. It's not the millions of keywords, but what do people want. That's what we as search marketers want to find out, what do people want? What's their intention or purpose?
JR:
Search intent is user intent, audience intent. I thought this was a pretty good overview from Yoast, who's got some really good content in addition to Clearscope. But search intent is more find, obviously, just to the search context of people going online and searching in their browser bar, searching in Google, or being to find what they're looking for online. The four types of search intent are informational intent, navigational intent, transactional intent, commercial intent.
JR:
There's plenty of articles written about this. If you search for search intent, they go into very deep detail about these, so I won't do that here. I would argue that, I think it was a couple of years ago, I had 80 million search or keywords that someone handed off to me. And I did this major scrape of Google, and essentially tried to extract all the unique features that Google has on the page. From People Also Ask, to images, to videos, to local, to shopping, to whatever. Any feature that they had on the page and just scraped those 80 million universal keywords and tried to put together essentially buckets.
JR:
The hypothesis was the construction of the features that Google provides on the page gives us clues to the type of intent that Google is showing. So obviously if there's a huge image block at the top of the page, then Google knows that people want to have an intent that they want to view images. They want to see things, they want to be inspired. So that came about to much of nothing, it was a waste of about several months, but it was fun.
JR:
But I think it did give me some ideas that people really have a visual intent sometime. If you're a designer, then you probably don't want to be buying something, you might just be saying, "I'm looking for ideas." So that's a different type of intent, I think, than some of the other categories. I think the idea of online versus local, Google says that with their... I can't remember the four words, but they do go no simple.
JR:
But there's definitely a difference between people wanting things online versus people wanting to get in their car and drive and go get something. I think rank tracking tools, this is something I have to teach every one of our analysts at LOCOMOTIVE, is that sometimes you're working in inches and you see keywords that have 400 searches a month and two clicks and you're ranking three for them. That is not real searchers. But you're in a competitive niche, and every one of the competitors in that niche are running different rank trackers. And those are impressions in Google. So sometimes your keywords aren't really useful keywords.
JR:
And then I think another intent is Google Translate. I think if you search for Pac-Man, Google has a Pac-Man game that comes up. But these things are people just want to perform some function, square footage calculated for a house. I don't know if that maybe that falls into informational, but I think it's a large enough category that it could be its own thing.
JR:
But I think pushing past intents, what if our goal is not to put search query into four buckets of intent, but to understand all the important types of intent, right? So you can see here search query, coda meaning, the meaning of the word coda. Coda Netflix, I want to watch the movie CODA. Coda software, to download software Coda. I went back and forth on this one, is that the right one? But if you're searching for Coda software, you want to go download or obtain that software.
JR:
So just learning how to really classify all these key into intent areas can have a lot of value for you, and really subsets a lot of the intent categories here into hundreds of thousands of additional intents that have value. A little diversion here, just because I like picking on Google sometimes, is that I really doubt that alphabet is what was, Alphabet, the company is deserves this much space for kids, and teenagers, and teachers, and parents, and all the millions of other people that are not looking for the company Alphabet search every day. I think that's interesting.
JR:
I think if I search for fonts, number one result is Google Fonts. I think that's interesting. I think this is more interesting, if you search for alphabet and font in Google Trends, maybe someone here knows type of precipitation is related to alphabet or Justin Bieber songs. I looked up Justin Bieber, Alphabet and I didn't see one. I wonder if Google's jacking with alphabets somehow and it's showing in Google Trends here that, this is just not aligned with anything here. Because they've made it their company intent. But anyway, that's not of my business.
JR:
So problems that intent-focus solves. I like the movie CODA. It's a beautiful movie. If you have not seen it, obviously everybody knows about it by now. But is really a beautiful movie and I recommend it highly. But I like the word coda because I think it's a really interesting word. If you look at the definition of the word coda, it's a concluding event, mark, or section. It also means Child of Deaf Adult. It's also an organization, Co-Dependents Anonymous, and an organization, Global Community of Healthcare Professionals. And it's a web development software, I believe for Mac, which is interesting.
JR:
But I think the thing that, maybe the reason SEOs have talked about intent so much is that they hate keywords. And keywords are not intent, they're just search terms people type into a browser and different tools throw us all these search terms back. Keywords are also super messy, just as a call out here, I do know that CODA is on Apple TV. That's just made a more compelling example. But anyone who's worked trying to pull Search Console data and make sense of it. And I think this is from AA traps here, but just the amount of garbage that you get when you actually get real user queries coming in and trying to parse those and understand those. This gives you an idea of how difficult that can be.
JR:
And there are a lot of keywords, again, from Netflix here. And this is obviously tools like Ahrefs and STM much don't cover all the keywords available on the internet or that people search for. Anybody that has worked on very large sites know that it's only a slice of the types of long-tail queries that you can actually see from data from Search Console. But it's still a large number, how do you make sense of nearly three million keywords and help understand that? That's the difficulty. And that's one of the difficulties that intent solves.
JR:
The keywords do reflect what users are searching for. So if you go through and just step through a lot of the different keywords that people are searching for, people are looking for movie details. People want to know where they can watch it, they want to know if they can watch it for free. They want to watch it on Apple TV. They're looking up information on who are the cast members. My daughter does this. Anytime there's a movie we watch, she's really into the people and the actors behind the movie. So she'll be going her phone and search cast members and just read that for 10 minutes. So she can understand who about who they are.
JR:
And then is the cast of CODA, Deaf, where they're all deaf cast members. I think that's an interesting question. But it's a pretty interesting... That's one of the things I really love. I think one of my parts of being an SEO is, I don't like a lot of, like the black hat stuff. I think my mission as an SEO is really about adding value. And I want to add value to users. When users type stuff and enter on my sites, I want them to get value out of that interaction. And I think understanding intent and understanding and translating what they're typing into what they're looking for is a really big area that I have a lot of interest in because our goal is to make sure they're satisfied.
JR:
I do also think that it's interesting when the meaning of words change. I did a tweet a couple of years ago now on how intents change seasonally. When a word can mean one thing at Christmas and mean something completely different in March of the same year. And Google reacts to that, "Why did my rankings go down?" Well, why people don't care about that anymore, they care about this other thing. So I'm just thinking of poor CODA here, that's probably like, "Yay. A movie came out with their name." And then they're like, "Well, why is our branded search dropping so far?" Well, it's because IMDB, Wikipedia, Apple, Rotten Tomatoes, it clicks them and they've gone down, they did nothing wrong.
JR:
It's just like Google wants to show good stuff for their users and what's interesting to their users. They don't want to make a site get clicks and have rankings. I wonder how CODA feels about it, I'd love to send them a message, and like, "What have you all been thinking with this?" That sounds really interesting to me. So point is, it is much easier to measure performance and change at an intent-level than a keyword-level. But there's also much more upfront work with it.
JR:
I would argue that it's also much easier to talk to non-SEOs, especially writers and marketing directors about intent rather than keywords. A lot of writers you work with, if you talk about keyword and then their mind is going back to all they've read about keyword stuffing and keyword density and all these really terrible things that SEOs have been known for in the past. And it's really hard to, I think, break that chain. So when you talk about intent, you talk about entities, you talk about intent satisfaction, I think that has a bit more better resonance to it.
JR:
All right. So limitations that SEOs have with an intent-focus. Ambiguous intent. So Google has to guess a lot, but one thing is that they're really good at it. I think there was a paper they published or research, they were published several years ago that talked about how amazing they are at the top million searches, especially really short-tail searches. And they're really good at those, they really understand those. It's the longer tail searches that they've had a lot of work to get better with, a lot of their different machine learning updates.
JR:
But the blocks to right indicate intents that Google thinks shooting by the search. If you searched office, it's not a lot of information. But they think, "Okay. You probably want Office, the software or you want to drive to an office supply store. Or you want to go online to this office supply store." And then down here in the corner, they have more of this information on sandwiches, maybe they mean an actual office.
JR:
So I think it's interesting here, if you were looking to see images of different office arrangements by this query. This would almost compel a second refinement, which is what we call crew refinement, where you go in and like, "That's not what I wanted. I need to add more context to this search." So you might search office images as your second search, but that would be a missed click and an impression for each of these results. That gets to lack of context.
JR:
So if you look at the words to the right here, coda, editor, Mac. You know what Coda is, you know that's the software. CODA, Rotten Tomatoes, you know that's the movie. Coda Conference, I think there may be two conferences, so that may not even give enough context there. And then I don't know that this one is, it was one of the search terms that came back. But CODA chemical, I don't know if there's a chemical company named CODA. But point being, is that this context here gives us information that Google, and we can use to infer what the meaning or what the entity behind this word is.
JR:
But that's not as easy when you have keywords like CODA reviews, or CODA cost. CODA cost could be the cost to rent the movie, the cost of the editor, the cost to go to the conference, or the cost of this company's chemicals. We just don't know, there's not enough information here. So search engines have context. If you searched for best editor for Mac, and then you looked at some different results and created an option set that you were interested in reviewing. And then you started going through the option set and then searched CODA reviews, then they could use that information.
JR:
They don't always, but they could use that information because they have your search history. Chat bots is a huge area right now of research and development. Chat bots use language models, and language models have this mechanism called attention that they can look at the past full history of your communications up to that point. So the chat bot says, "Hi," you say, "What's the best editor for Mac?" The bot says, "Coda." And then you ask, "Can I get some reviews for Coda?" And then the bot says, "It's a good text editor." So it's using the attention for your previous entry of what is the best editor for Mac to infer the intent of Coda as a text editor, instead of just taking this at face value.
JR:
I thought this was interesting, I think even Google misses clues. You have defined Coda here, and then you have defined CODA, all caps. I almost expected Google to return a different version of this, but they didn't. But I think it shows that Google has a way to go. And perhaps, maybe they lowercase everything when they ship it. I don't know. So a lack of context in keywords makes leveraging more advanced NLP tactics like named entity recognition, it's called NER. Almost impossible for terms with limited information.
JR:
I think this is a really good example here. US Steel reviews. You may be aware of US Steel as a company. But this also could mean US, the country, Steel reviews. I have to pause, one second. Apologies, I'm living with dogs and working from home. So the lack of context here is something that you almost have to go out and get additional context from somewhere else. So in this case, if we're presented with US Steel reviews as a keyword, and we want to know whether that means US Steel the company is an entity, or this is someone looking to understand reviews of the steel industry in the US.
JR:
We actually have to use third-party data who can give us an understanding from their users about the types of content that their users find interesting with respect to that. So you can see here that if you do a search for US Steel reviews, all the entity information on this page is talking about US Steel as a company and not US-based Steel company reviews. So how intent aligns with your journey and funnel. I've been doing a lot of research recently around chat bots, chat bots are really, really interesting.
JR:
One really common research area in the machine learning community around chat bots is the idea of intents and slots. So you can see what they call an utterance, which is, "Are there any flights from Long Beach to Columbus on Wednesday, April 16th?" So a chat bot really needs to understand, "Okay. What are they looking for," right? And also to be able to then pull pieces of information from that utterance so that they know and can give to a machine to say, "This person is looking for flights from Long Beach to Columbus on the state."
JR:
So the intent is what the person is looking for, so it's labeled this as a flight. And then slots are the semantic entities related to the intent. So that's the city name, and then date information. If you were developing onsite search or chat functionality, this is what it might look like. Now, going back to our example from coda, watch CODA on Netflix. The intent would be to watch a movie, and then the movie title would be CODA. And then the service would be Netflix, to be able to compartmentalize this query.
JR:
We can align our sites intent using search queries in the same way, where to watch CODA movie, watch where? Kind of thinning this on how to watch with the conflation of where. CODA, Netflix, I want to watch it on the exact service. I'm looking for movie trailers, and then I want to watch it free. Free is obviously a different category of intent from, "I want to watch it and I'm willing to pay." So obviously that needs to be handled a bit differently.
JR:
I thought this was really interesting, but then AI has their GT... I always get it wrong, GPT-3 model, which they now allow editing so that you can essentially give it some input and say, "Fill in the missing data." So in this case here, we gave it some different intent classes. Maybe watch where, maybe watch exact, maybe movie trailer, maybe watch free, which were the same ones from here. And then I asked it to fill in the movie intent class and the search intent class.
JR:
So I think the search intent class is debatable, but it did a good job of classifying the movie intent class. So this is what... And this is one of the proposed solutions using language models for the problem of intent and slot classification. There's also a lot of other languages, or a lot of other model types that are being used to tackle this. I thought this was also really interesting, is that it's really fun to play with other types of query classifications.
JR:
Just going back to the intent and slot example, "Netflix movies to watch," it inferred it was informational. The journey stage is awareness. The intent is the repetition, geography is national, that makes sense. The brand, the entities associated with it, and then key subtopics. So we haven't played with this a lot, and I think at a keyword level G, the OpenAI, Davinci model would be pretty expensive to go to that level. But it's still interesting, I think, to play with and see the power of that model.
JR:
But so this is a model called NeMo, which does the same thing. You essentially give it a list of queries or utterances and give it some classifications of those queries as certain types of intents, as well as certain of the keywords or individual words in those phrases as certain types of slots. And it will actually help categorize for you on never before seen data. So this is a much lower cost alternative than going with something like OpenAI. But obviously, the setup and training of the data is a bit more difficult.
Recommended reading: Why You Should (and Shouldn't) Use AI Content Generation
JR:
But I think just using... And I try to put everything here as a really toy example of a lot of this, just to get the idea across. But let's say I worked for... I conflated Netflix and Apple, again, here, but let's say that I worked for Netflix and CODA was on Netflix, and I say, "Where to watch CODA, where to watch CODA free?" I do some searches and I find out that I can't watch CODA for free, I actually have to pay, or maybe I can get a free trial for the service. And then I might do a navigational search back to that service. And then maybe I'm, again, looking for cost to the service and then maybe free trial and whatever that journey looks like for them.
JR:
And then you're essentially mapping these back to certain intents. And I think the interesting thing here is the aggregation. So for one movie, it just doesn't make a lot of sense, but when you have hundreds of thousands of movies or thousands of movies where you're not specifying it in an intent tied to a certain type of specific movie, or maybe you have actors, or maybe you have any page on your site that have a lot of product landing pages that are really homogenous in what they are. They're more than likely going to be a lot of similar intents that tend to happen around those types of pages.
JR:
So this really gets interesting at scale, where you can see how you're performing and then align a lot of these intents to this specific, not only visibility bucket, "How well am I performing for this category of intent? What journey stages is this intent? What funnel stages is this intent?" And really aligning it with intent in search query. But using this is the glue that's holding everything together.
JR:
This is a pretty small section. But one thing we've done is to build a pipeline for essentially getting new versions of keywords. Cleaning the keywords that showed in some of the Netflix examples, you saw punctuation and really goofy spellings of different things, and just getting rid of a lot of that. And then doing, not only clustering, but intent and entity classification. This is doing a lot of work here in this scrap, in this chart, because this is a huge step to get this done.
JR:
And then getting that into things like BigQuery and using either Google Data Studio or Data Studio with Funnel IO to be able to connect to BigQuery, doing some blending on new data and then reporting on that data. So this is a long pipeline to develop with a very heavy stage here. But it's worth its weight in gold, especially if you're working on larger sites where you can have some of the changes, and new releases, and movies, or things that people haven't really seen before. You can already have your intents to find and see how those intents align to new data.
JR:
This is looking at for a pretty large site intent coverage at scale. And I like throwing it on this graph this way, because these intents right here are ones with high impressions, but low clicks. You can also use something like bubble size to infer, either click through rate, or if you have some data set that aligns intent to value, give essentially a look up of essentially how valuable is intent from a revenue perspective.
JR:
But this does give you a way to, A, visualize all the intent that your site is surfacing in one view. But also intents that you can directly take action on to move these in a direction and take advantage of the impressions that you're getting. Another big area for us of after you have put together and really categorized a lot of your keywords is understanding how those intents align with certain types of pages across your site, or maybe align with the wrong types of pages.
JR:
I think getting back to the earlier statement where I don't like where I see my job as an SEO is about adding value. So I see we want to increase traffic, but I think more important, as important as wanting to increase traffic is we want to get people going to the right pages on the site that satisfies their intent. It's not good if you have a page that just has a lot of content, but is a terrible experience for users, is ranking really well and getting a lot of traffic. And maybe another page with less content isn't doing as well, but that is a page that would be a lot more helpful to users. Understanding that at scale, I think, is really difficult. And an intent focus, it gives you, I think the level of granularity needed to be able to make those decisions without getting lost in the weeds of millions of keywords.
JR:
All right. So strategies for finding new intent, whitespace areas to cover. I manage to be able to get through this in six minutes time. So the first is your standard content gap. Ahrefs has a really nice content gap built into it, where you can put your domain as well as three to four competitor domains. And they'll essentially tell you where you're missing coverage. I think it's important to note that you can also do this on a page level, or you can do it on a subsection level.
JR:
So if your competitor has a lot of content, that's not really relevant to what you're doing, the common way to handle that is to put either a section, just target a section of the competitor's site, but you can do it. In this case, I've done it at the page level between IMDB, Wikipedia, Apple TV. And you do see some interesting ones here, like, "Where was CODA filmed," right? Film location could be something that IMDB adds to as an intent to all of their movies. That one thing could be a large net win for IMDB, just with that one additional feature added to their pages.
JR:
One note here is, I wish Ahrefs would include a search feature filter here to exclude sitelinks. Sitelinks typically denote navigational queries. And I like to stay away from navigational queries if I can. Since those tend to rank higher and be less likely to drive traffic than others. So that would be a good add if anyone from Ahrefs is listening. But here's another one, this is Semrush, same thing, you can add in a page or a domain or a subdomain or a sub folder, and then several competitors. And then they'll give you essentially where you're missing compared to your competitors.
JR:
I like, as I said, Ahrefs version better for me, but I do like the fact that Semrush has a nice pivot that seems really helpful for SEO and SEM teams. In addition, I love whoever made the decision at Semrush to make their API a lot more accessible than the large charge of $500 a month so that agencies can have more self-service access to it. Another area is reviewing aspirational owned keywords. So Google is the world's best relevance engine at knowing how good content is to keywords or to search queries.
JR:
So there's a lot of clouds of just misses outside of the top 20. You're not really relevant for that, like a lot of those people are more relevant for that, but you're kind of relevant for that. So I think looking through, and you can see here, I think, I don't remember which site I did this on this page. I did this on, it may have been IMDB, but I just looked for positions 50 to 100, with a max volume or a minimum volume of 300, just to get a dump of keywords that I could look through in that category.
JR:
And you can see there's 84,000 keywords, position 50 or higher that are somewhat relevant to this site, but they don't have content really targeted for. Using a bit of Python, there's a library called NLTK that has, or maybe it's SACE Learn that has a function called countback browser and N-grams. And I won't go into a lot of detail about N-grams, it takes a phrase and... If you have the phrase, "The black cat," it'll return as a one gram. The black is the two gram and then the black cat is three gram, pretty simple.
JR:
But you can essentially throw in 1,000, 10,000, 80,000 keywords. And just ask it to return back to you, in this case, just the top instances of two or two, five word N-grams found across all of those keywords. So in the top 50 example I showed before, we showed people looking for the Blue-ray version of it, that's a potential additional intent where they can watch the movie for free. The streaming service that a movie is available on, where you can watch it by location, maybe even song lyrics associated with that movie or songs in that movie.
JR:
So just from a really quick dump of IMDB here, and then just one tool count vector as you're able to just really quickly parse through hundreds of thousands of keyword and find some unique categories of intent that you could maybe add to your intent matching data to this point. And obviously either add new content or features to existing pages or to even generate brand new pages to targeting those intents.
JR:
Another area, there's a couple of tools in the Python ecosystem called Flair and SpaCy, this is one of my favorite things here. But again, taking, hundreds of thousands of keywords that you find, and then running it through Flairs, NER tagging, named entity recognition. This second one here essentially filters out anything lower than one percentile or anything above the 99th percentile, which is really great at getting rid of misspellings. Like the Netflix example, that are really infrequently in your data set, and things like stop words, the two that don't really add any unique value to the intents.
JR:
And the final thing here is lemmatization, which takes phrases like providers and makes them provider, so that you're not looking at duplication of those types of queries. But you can see on the right here, what this does, which is internet provider, location, city, internet provider, location, which this would match across a bunch of keywords saying internet provider Raleigh, internet provider, Charlotte. But you're essentially able to just distill hundreds of thousands of keywords down to a much more manageable set of intents by doing some of this analysis first. And you're just not going through page after page, after page, after page of the same types of keywords.
JR:
So in terms of mining for new intent opportunities, we like the API ValueSerp, it's very cheap. And I like to do a six degrees of Kevin Bacon strategy, where I essentially give it a set of our base keyword. So these are the keywords that I know about. So these are the keyword archetypes that I know about that I want to rank for. And then we'll actually just crawl Google for those keywords and pull back related phrases, though the related phrases into a bucket, and then crawl all the related phrases, throws those into a bucket and just keep doing that several times.
JR:
And at the end of that, you can come back with hundreds of thousands of different queries in an hour. That some go on tangents, but a lot of them are really relevant or can give some ideas of some new intent areas. But yeah, this is really recurse related, the depth is four, I want it to recurse those things four times that it finds. And then, yeah, all of a sudden this was from one keyword, but we pulled back a list of recursing four times, I think it was five, 600 different related queries from just the term coda.
JR:
So really easy to find a lot of related, not only searches, but you can do the same thing for People Also Ask Questions. People Also Ask Questions are equally impressive. So this is what one of our... We either report these to BigQuery or use using Google Sheets, but we'll get all of our keywords here. And then we actually manually... Well, we have internal processes that do a lot of the NLP, the name entity recognition, and then do some clustering to be able to come through here and assign categories to things.
JR:
And the categories are essentially the intent here. So landscaping Dutchess County, landscaping location, landscaping Niceville, Florida, landscaping location. You can see how all these different searches here just rolled up into this one intent focus here. Then the goal of this is to get from go looking at hundreds, or thousands, or tens of thousands, or hundred of thousands of keywords. And looking at 100 intent categories, looking at 110 categories, and how you're performing, and how they align the journey and stage, and everything, is heck of a lot easier to manage than hundreds of thousands of keywords.
JR:
This also gives you a set of labels here. If you take the time and very rigorous with going through and validating, I've shown some of the examples before of how NER can get it wrong. So actually going through your data and making sure that everything is labeled appropriately before taking it to some of the machine learning pipelines like NeMo is a really critical step. But another interesting area is that after you've labeled a lot of your intents into your different intent classes, you can actually run tools like, again, NeMo to give you predictions, like, "What is a certainty with which it's classifying this intent?"
JR:
So CODA movie language is movie information, Coda for windows is software. And it's giving here the percentage of certainty that it thinks it's making that prediction with. So obviously higher certainty means it's a good classification, low certainty, either means that it's like a really ambiguous intent. If I just put in DA, every one of these classes would be low certainty. But it's also an opportunity, that maybe this is a brand new intent.
JR:
So actually there are other research pipelines going through and just trying to look at bad classifications and intent classifications. And really using those to mine and look at different new intent opportunities for chat bots. But here we're taking that application and moving it to search space. That's it. Questions?
Bernard:
JR, that was amazing. So much good stuff there. And so many resources that I've been kicking into the chat as you've been speaking. So we'll definitely sum all of those out in our recap email. So you don't have to jot those down or open a bajillion tabs and think that you're going to figure those. But I have a question to kick off all of the questions. How much do you think this costs at a keyword level?
Bernard:
Obviously, we do a lot of natural language processing entity stuff here at Clearscope, and it's not cheap. So for 1,000 keywords of class classification, for everybody who's looking to do this manually by stitching together all of the tools that you are pointing out, how much do you have you gotten that cost down to?
JR:
I think it really depends on the type of site that we have. So obviously if it's a larger site with larger budgets, then a lot of this stuff makes a lot more sense. But I think we run, I think, anywhere from all of our classification and resources are roughly $1,000 a month. So I think within that area, and that's using a lot of these types of frameworks over, not a ton of clients, we're a smaller agency, maybe 10 to 15 clients.
Bernard:
An anonymous attendee asks, "Did JR intentionally coordinate the color of his shirt with that bubble graph?"
JR:
Oh, maybe. I don't remember where the bubble went.
Bernard:
Yeah. Maybe that bubble graph.
JR:
I don't know, maybe.
Bernard:
Maybe. It's all right. All right. I think Derek asks a very interesting question, "How could a tool integrate all these tools to lower the bar for less technical SEOs or SEOs that don't have as much engineering capabilities?"
JR:
That's the thing that I wanted to call out more than anything, that's one of my most hated things with tools out there right now. All the way from Semrush to Ahrefs, we're still on this keyword area. And then there's all this talk about, obviously, like Keyword Magic tools, were a big step. But I don't think anybody is really working hard on just companies like Ahrefs and Clearscope, I mean, Ahrefs and Semrush have the resource, and they could do more.
JR:
I think there's open intent data sets right now, where companies are trying to do these global intent data sets. And I think tool companies probably should do a lot of this and integrate a lot of this. But I do think it may take some working together and it would take some considerable resources. But Semrush has intent classification right now. I don't know anybody that's used it, I think it's pretty okay.
JR:
I think it misses a lot, but they're obviously going in that direction and I would love to pull a report from Semrush and these are the 40 or 50 intents that your site's driving. Rather than, "Here's the 2.4 million keywords that your site is driving." I think with all the research that's out there right now, I don't think that's that far away.
Bernard:
Yeah. Yeah. I would agree. Because machine learning and entity classification I think is so new, that there's a lot of people running around with all these different models. And as a result, you don't have any common consensus on, like, "Movie. Watch. Where?" Is one model. Whereas you could say that that's a research intent, which is another model. And without, I think, common consensus amongst all the different libraries, it's going to be hard to have a cheaper, more streamlined methodology.
JR:
Yeah. Great.
Bernard:
Yeah. Emily asks, "This is a question for somebody who can answer. If we use WordPress to post do keywords added as tags, help a bump of Clearscope score." So I guess I'll answer that, they would bump your Clearscope score because the tag would show up as text on the page. And then our understanding currently is that Google is reading all of the text on your page. And we believe that they're using some sort of a scroll depth thing to basically figure out that something that you put higher up on the page, that takes up potentially more screen real estate, is likely to be more important on your page than something that's lower on the page.
Bernard:
And so that's why you see a lot of people try to stuff entities in headers or nav bars, rather than stuffing them in the footers. But yes, we're right about that time I would say, "In lieu of having a tool basically solve this whole magic of taking millions of keywords and boiling it down to 100 to 200 clean to view intents. That's why agencies like LOCOMOTIVE exists." Obviously I can think of no better agency to hire, to do a lot of this machine learning classification things. Especially if you have millions or hundreds of thousands of landing pages to look at then hiring LOCOMOTIVE.
Bernard:
So definitely LOCOMOTIVE is your bet. I'll put their contact details in the recap email that we send out sometime soon. And JR, thanks so much for this amazing presentation. It was full of both very nerdy, but very relatable challenges and problems that I think a lot of us are struggling with SEO. And it was just brilliant to walk through those different examples of ambiguous search intent. And basically trying to complete that user journey. So thank you so much for the amazing stuff.
JR:
Awesome. Yeah. Thanks for having me. Sorry about the dog problem, but she's happier now. And really looking forward to seeing you all's new releases at Clearscope. You all are doing a great job.
Bernard:
Awesome. I have one actual question that just came up. This is an interesting one. Any insight on how to adapt this kind of strategy for prohibited queries by Google? You know these as your dangerous goods and services, basically cannabis. Children related things have recently been bucketed into that. Because keyword search volume data for, well, really a lot of the main tools out there are lacking for prohibited stuff.
JR:
Not really. We aren't really in those areas with a lot of the work we do. So I don't have a lot of feedback on that.
Bernard:
All good. Yeah. Me neither. I know it's a big problem with just keyword that Google say is prohibited. And if anything, Google is getting way more stringent on handing out that data. So they just had an API update happen, where, if you had standard access before, they clamped down even harder on what the limitations are that you could do. And I imagine that search volume data is going to become less and less accessible to everybody as the years go forward.
JR:
Yeah. I can see that.
Bernard:
Yeah. All right. Well, thank you so much for tuning in, JR. I'll follow-up shortly, and take care.
JR:
Thank you guys.
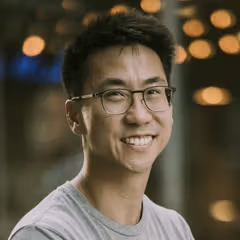